The Automation Experiment - First Report
28 Feb 2016For those who have been reading regularly, you might know that I am pretty interested in home (or in my case, dorm room) automation. In my sophomore year (last school year), I began work on automating my dorm room here at Rice University. In a few paragraphs, I will introduce you to Domo — named after the Spanish word for butler mayordomo and the Esperanto word for house domo — the latest version of RRAD (Rice Ridiculously Automated Dorm), but first some background.
The original system allowed control of a series of several LED strips, including the ability to toggle the power on and off, and change the light colors; it also had the ability to power a fan in response to room temperature using a home-made relay box which independently switched 120 V to two power outlets. I designed a very nice web control panel, complete with the ability to monitor, control, and review logs from the automation system, as well as mobile integration for easy control. I submitted this original project to the Hack-a-Day Prize that same year and was awarded quarter-finalist; my work would later be featured in an article on the website.
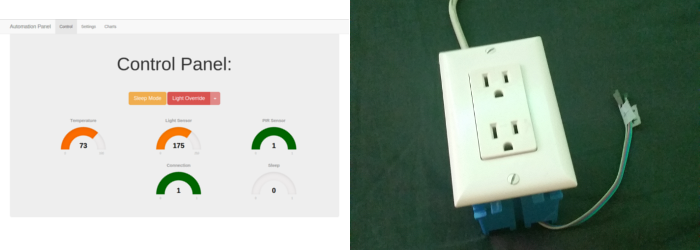
A screenshot of the web interface, and a photo of the relay-controlled power outlet box - of my own design and construction - from automation system version 1.
I had one other major inspiration for this project: in early January, I published a post in which I discussed my vision for an advanced automation system in response to Mark Zuckerberg's announcement that he would spend this year developing his own intelligent home. Now, I wish I could build the system that I have described, however I do not have the time, nor money/resources. I am a Rice University student living in a dorm room, so I am on a student's budget, there is not a huge amount I can modify, and I am currently spending much of my already limited free-time studying for the MCAT. I cannot drill into the wall, nor safely manipulate the electrical systems/switches. As such, I have had to be creative and efficient in the ways in which I automate the various systems. Despite all of these challenges and limitations, I was determined to try and build a voice-based automation system with the resources I had available (in other words, as cheap as possible).
Before I began working, I had to do a significant amount of research on the mechanics of voice recognition software, and the various open-source solutions currently available in order to figure out how best to accept voice commands into my system. There are numerous solutions available for converting speech to text (STT), but the majority are web services, which require you to send your audio data over the internet to a 3rd party. I was initially determined to utilize one of the two major open-source, offline STT softwares: PocketSphinx and Julius; because I was concerned about privacy and hitting quotas for the services. I eventually did give up on using the offline STT softwares as they are not yet sophisticated/mature enough for my purposes in this project; I now use Google's STT service.
For my first attempt at voice recognition, I downloaded and setup Jasper, a software package which can utilize either of the above offline speech recognizers (as well as a number of web services) to provide keyword activated ("hey Domo!" or "hey RRAD!"), always-on voice recognition. I settled on using PocketSphinx for STT because it required significantly less configuration and training — though there is still quite a bit — however I never really got the accuracy I was hoping for. There is a piece of software produced for KDE called Simon which is intended to permit training of PocketSphinx and Julius, but I was unable to figure out how to work effectively with it. I was also not a big fan of Jasper, as the code is not easy to work with, and the developers focused on supporting numerous speech-to-text services rather than providing a single useable workflow for always-on voice recognition; ultimately this first experiment was a failure.
After my disappointment with Jasper, I turned to working with another piece of software called Blather which I found to have a much more manageable code-base, and which provided much better support for adding new voice commands. After spending some time manipulating Blather's code (written in Python), I got my system up and running! I added my own text-to-speech commands to make my creation talk using espeak; and I established serial communications with an Arduino which I use to send infrared commands I copied from my IR remote to control my LED strip (Python code & Arduino stuff). Version 2 of the system wasn't perfect, but it worked and I was ecstatic!
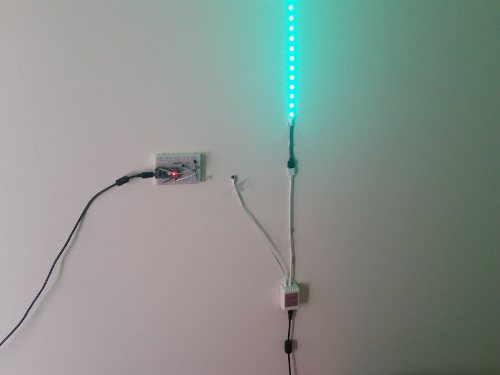
Here is the Arduino system I am currently using to test my setup; it consists of an IR receiver to decode the remote control, and an IR led operated by a small NPN transistor (supplying 5V instead of the 3.3V from the digital pin). It is placed next to the receiver in this image for testing purposes, but by no means does it need to be this close. It is currently sitting 3 to 4 feet from the receiver, and works around 90% of the time.
Even though this second iteration of the system was functional, I was not satisfied. The experience just wasn't all that I wanted it to be; primarily, the accuracy of recognition was far below what I considered functional. I briefly switched to using the free, online speech recognition service Wit.ai for my recognition, while still using PocketSphinx for my keyword recognition (so most of the time, I would process my audio data offline rather than sending it to an online service), but again, accuracy was not good enough (you can find the code for this iteration of the project here). Ultimately, my problems came down to the fact that I did not have a good way to record voice commands spoken across the whole room because my microphone was not good enough. I had to seriously reconsider my approach.
In my next post I will talk a bit more about the work I have done since version 2 to continue improving my system. I am now on version 3, having switched my approach once again, this time leveraging the speech recognition capabilities in my Pebble Time smartwatch, and the latest generation of web browsers to receive commands from any number of devices connected to my secure network (including my phone and computers). This newest approach has had outstanding results; I am content to using this means of voice recognition and simply add on new hardware features.
Below is a brief video demo of just a few of the capabilities of the latest system. I will write another post soon in which I will discuss how I finally created a successful version of my system, thanks for reading!